Interaction Surfaces explained: Why Context is Everything
Christoph Mülligann, Chief Innovator at Geolytix, takes us behind the curtain of developing Interaction Surfaces and gives us his unique take on making sense of mobile data.
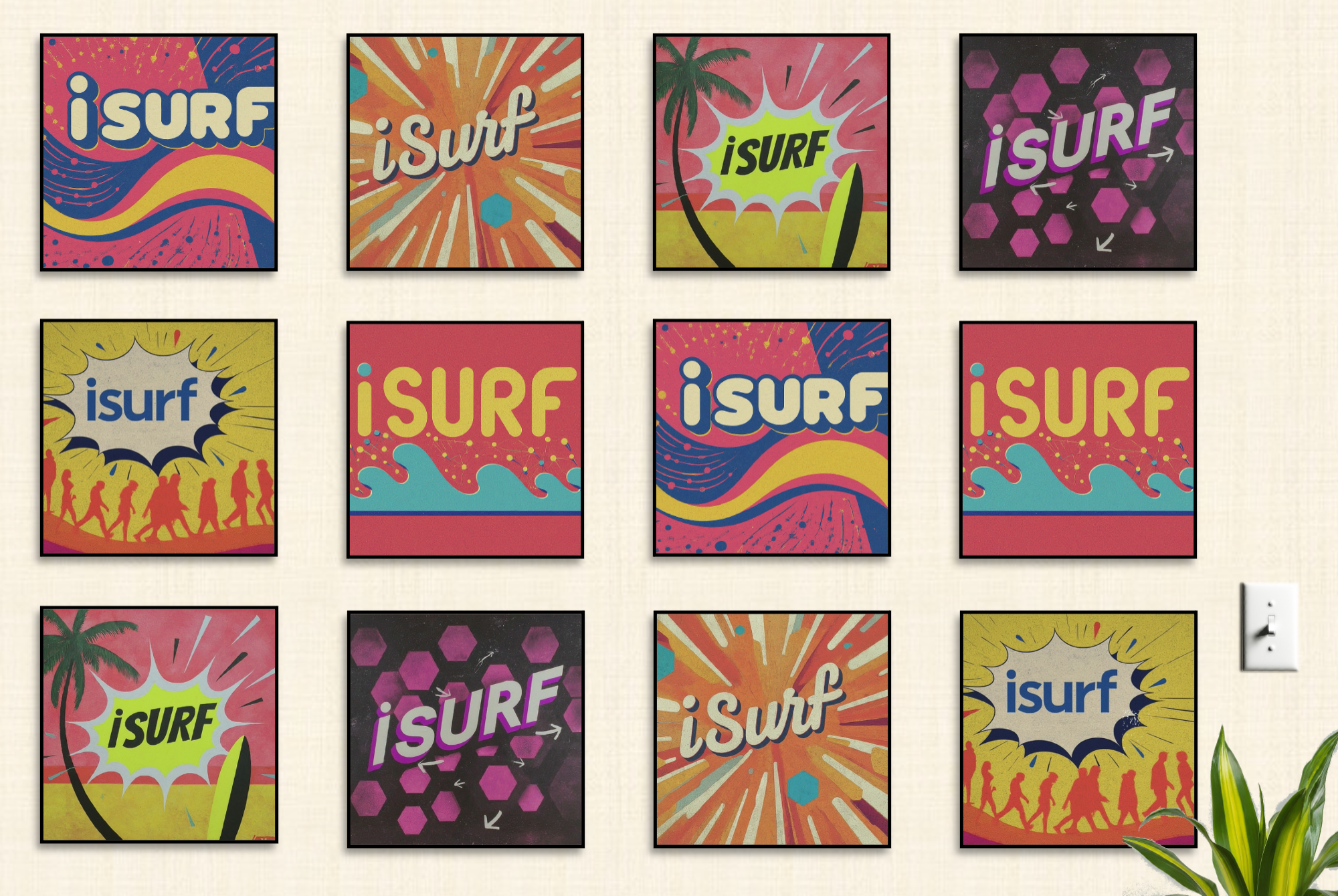
As one says*
The problem is, we blinked.
And now we’re left wondering how the arrow found its target.
And how the eating habits of certain holometabolans found their way into this blog.
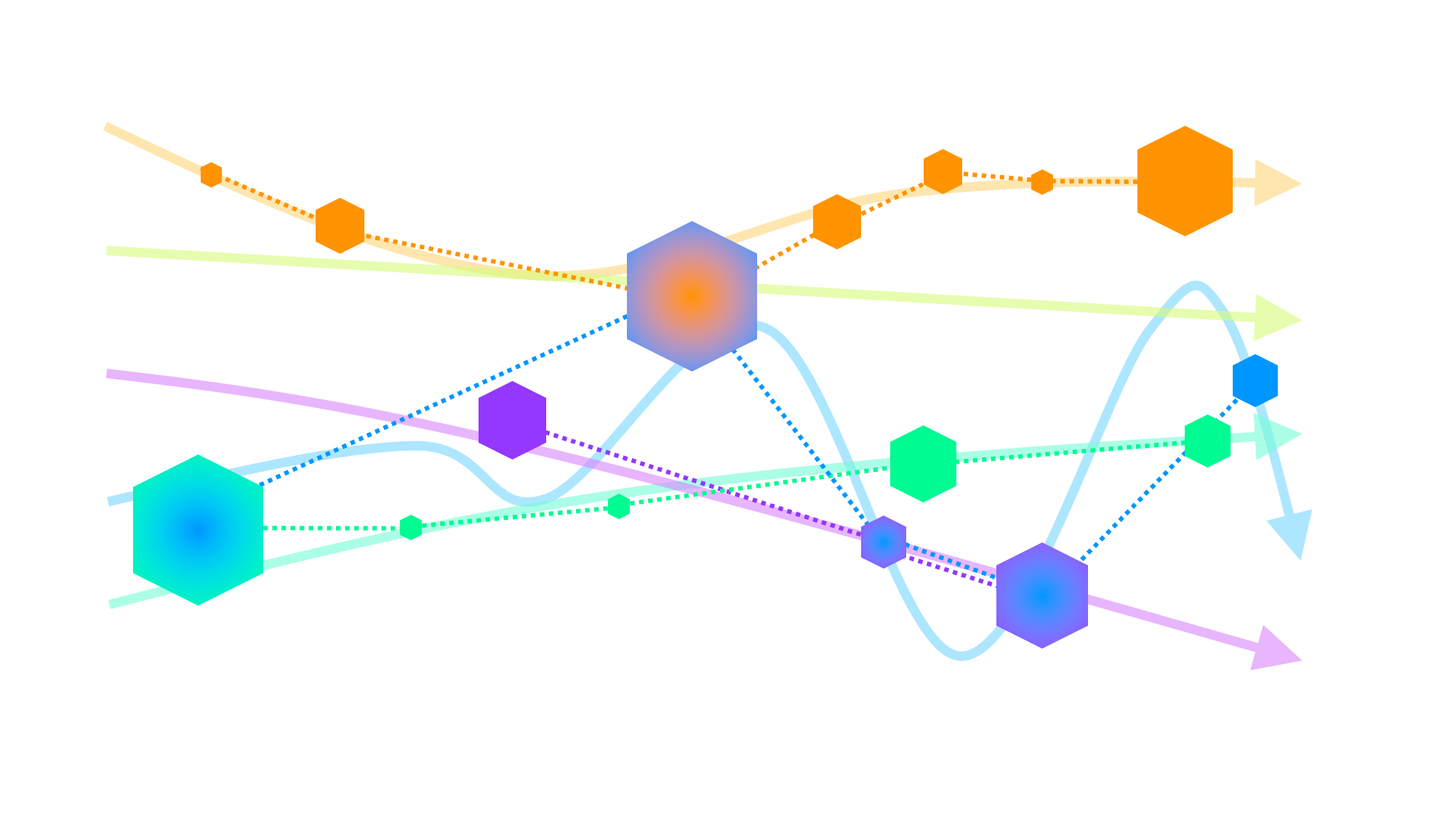
Whether you are analysing large amounts of ping data from mobile devices or the connection between a blog’s leading quote and its title, context matters. When it comes to Interaction Surfaces, without context-awareness in fact we would not have a product. Here is why:
Mobile data is a sample of real-world movements. But it is not only a sample in the sense that devices represent a subset of the general population. On the individual device-level, the discrete data points - or, as we call them, “pings” - are also a sample of a continuous path through space-time. Both are a necessary requirement for the safe consumption of such sensitive data but equally present a challenge for the interpretability of the data on an aggregate level. When developing interaction surfaces, we embraced the opportunities presented by the latent contextual information in the raw data instead of “aggregate first, worry later”. The following are some highlights from our methodology where this approach was critical.
From Fuzz to Focus
Let’s start with locations. In a mobility data set, they are reported at different granularity depending on the data capture method. That granularity is sufficiently high for many aggregate use cases. It may produce some ambiguity, however, for speed estimates, which are essential to separating pedestrian from vehicular devices and make sure we are always looking at the audience most relevant to our clients’ use case. By comparing slowest and fastest scenarios given a particular granularity, we can determine whether devices are in walking mode or not with relative certainty. Think of it as a vote - if both scenarios agree on the discrete outcome, numerical fuzziness is irrelevant. Where the vote is split, we can make use of the trajectory context to pick the most likely classification. As a consequence, our devices don’t flicker between walking and driving. We preserve valuable co-occurrence information of walking locations and our interaction surfaces are all the better for it.
At this stage we have only looked at a device location in the context of the device’s entire trajectory. For the final two points, it’s all about a device’s movement in the context of every device’s movement - call it “swarm intelligence”. (Yes, I have finally justified the fruit flies and the bananas - thank you for bearing with me)
Beam me up, Scotty
Unfortunately, teleportation is not a thing (yet). That means in the real world, in order to get somewhere, people need to go through somewhere else first - regardless of the scale at which you define a place. But, due to its discrete nature, even the densest ping data set won’t record how devices enter or leave a place at any spatial resolution.
By quantifying what that leakage is at the fixed resolution we deem most valuable to report daily pedestrian interactions on, we can manufacture a dataset where everyone - no matter how often they ping - comes from and goes to somewhere (we do exclude proper dwell locations like home, work, school, etc. for privacy reasons). It makes sure the values you see in our interaction surfaces are not only meaningful relative to each other but can be interpreted as actual probabilities.
Here, There and Everywhere
So we have identified which devices are pedestrians. We have made sure they don’t just zap around. We have processed our co-occurrence probabilities. But we are still looking at each pair of locations separately. What a waste! We might not know the likelihood of someone going from A to C. But we do know the likelihood of someone going from A to B and the likelihood of someone going from B to C. Cascading probabilities allows us to enrich the interaction surfaces data and give you more insights for a single location. I would not recommend trying this at home though and in fact I am contractually obliged to mention there is still a lot of AI and ML involved in this process.
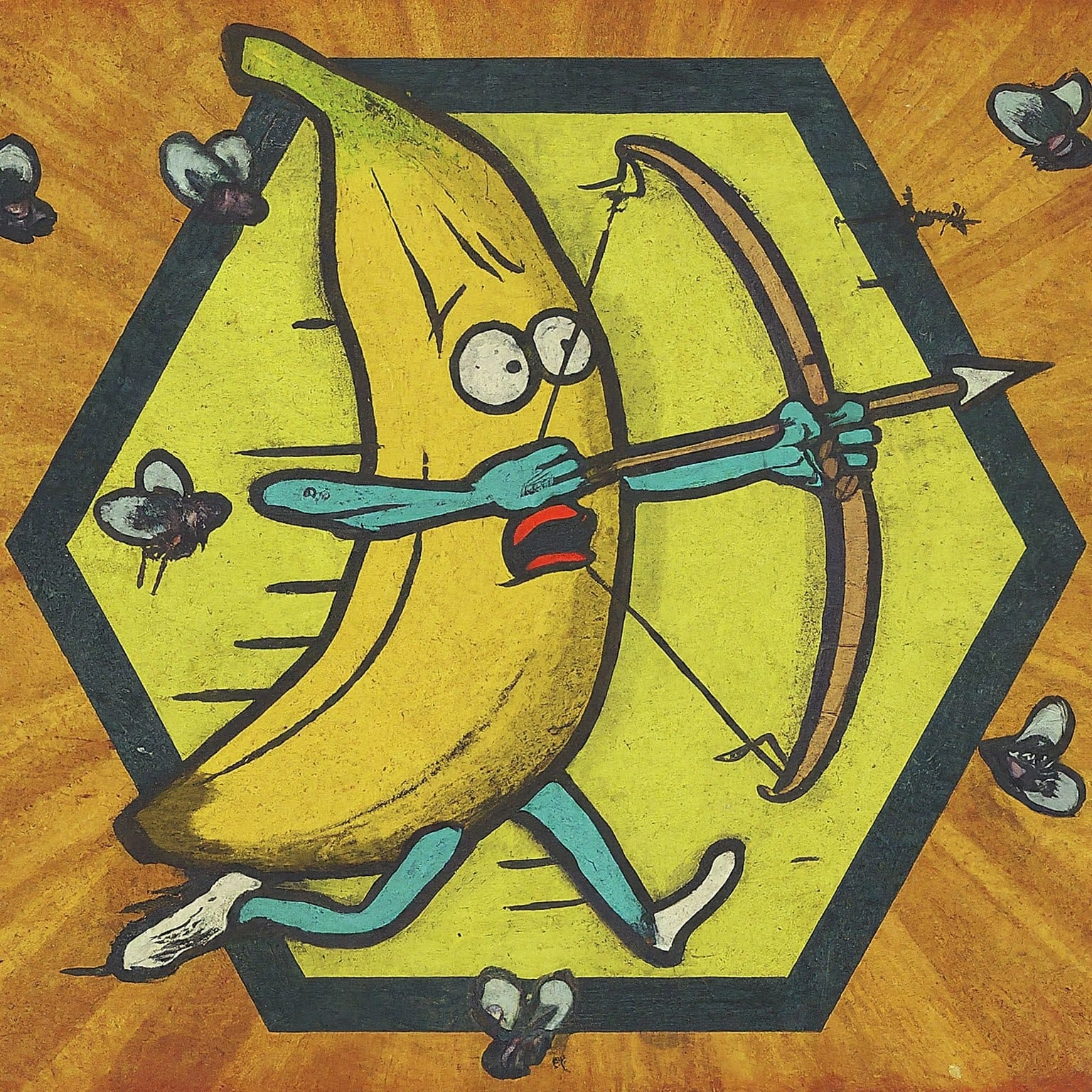
I hope you enjoy the product 😄
Author: Christoph Mülligann, Chief Innovator at Geolytix
*authorship contested
All artwork by Google Gemini